The Future of AI on the Shop Floor: What’s Next for Smart Manufacturing?
AI on the shop floor enhances human expertise, solving waste, downtime, and skills gaps. Discover smart manufacturing’s next phase.
Manufacturing expertise has always been a blend of human intuition and technical precision. Today, Artificial intelligence (AI) has the potential to amplify both. While it cannot replace the machinist who senses a misaligned gear by sound or the quality inspector who spots a subtle weld flaw, it could help turn those instincts into scalable, systemic intelligence in the future.
The Future of AI on the Shop Floor Summary
- AI could enable real-time adjustments to maintenance, production schedules, and quality checks as its integration evolves.
- Collaborative tools have the potential to bridge experience gaps while complementing human expertise.
- Future modular integration could allow AI adoption alongside legacy equipment.
- Sustainability gains emerge from optimizing energy and material use.
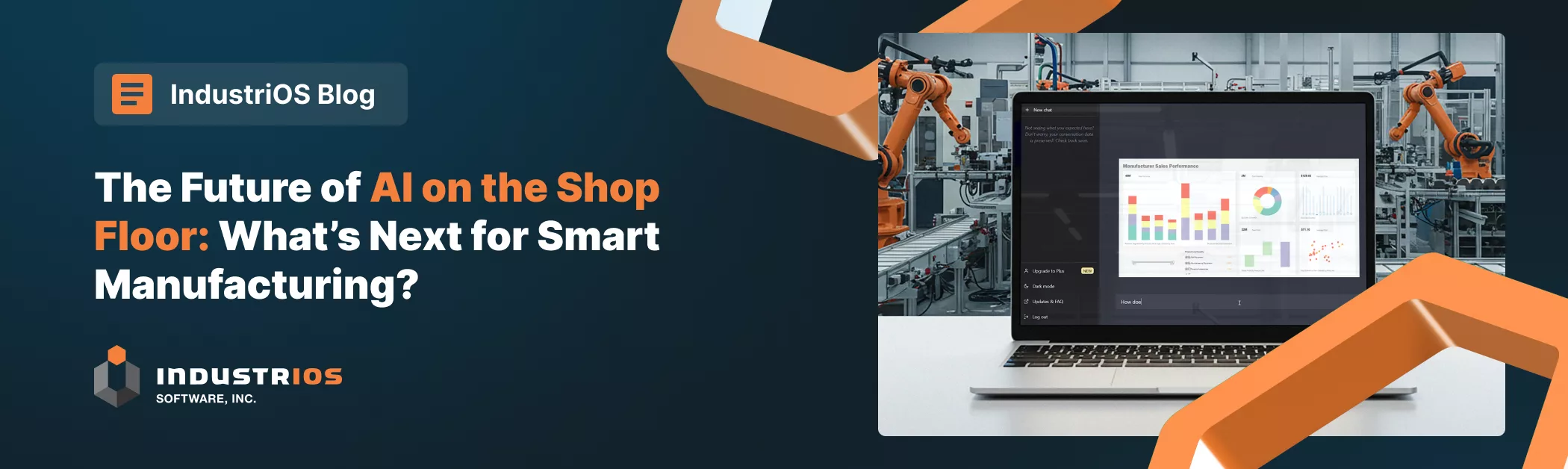
Maintenance That Speaks Volumes
Machines communicate through data: vibrations, temperature spikes, energy draw fluctuations. Traditional maintenance schedules ignore these signals, relying instead on generic timelines. AI could eventually interpret this data in context, factoring in variables like production intensity and environmental conditions.
Hydraulic presses in high-humidity environments, for instance, wear differently than those in climate-controlled facilities. AI models trained on these nuances predict failures specific to each machine’s reality. Technicians receive prioritized alerts and not just “bearing needs replacement” but “replace bearing X on press Y before the next high-volume run.” This precision turns maintenance from a cost center into a strategic asset.
Scheduling That Bends, Doesn’t Break
Static production plans assume ideal conditions: no delays, no shortages, no emergencies. Reality is messier. AI-driven schedulers could one day ingest real-time data—inventory levels, supplier delays, machine availability—to rebuild workflows dynamically.
When a resin shortage hits, the system reorders tasks to prioritize products using available materials. It batches similar orders to minimize tooling changes or shifts energy-intensive processes to off-peak hours. These adjustments are calculated against multiple objectives: hitting delivery dates, reducing overtime, or cutting energy costs. The result? Production lines that adapt like living systems, not rigid assembly chains.
Quality Control Without Blind Spots
Human inspectors excel at judgment calls but struggle with consistency across thousands of parts. AI vision systems have the potential to fill this gap by scanning products at resolutions and speeds beyond human capability in future implementations. They spot micron-level cracks in turbine blades or inconsistent textures in painted surfaces, flagging defects that escape the naked eye.
The true innovation is collaborative learning. When a veteran inspector overrides an AI defect flag, the system recalibrates. Over time, it aligns with the team’s tolerance thresholds, reducing false alerts without compromising standards. This feedback loop turns sporadic human expertise into institutionalized precision.
Capturing What Retirements Take Away
As skilled workers exit the workforce, they take decades of tacit knowledge with them. AI tools could preserve this expertise through interaction. For example, a milling machine operator might explain how to adjust feeds and speeds for a brittle alloy—a process that future AI systems might transcribe, structure, and convert into step-by-step guidance for new hires.”
Augmented reality (AR) takes this further. AR glasses overlay machine-specific instructions during setups, adapting to the user’s skill level. New workers see granular checklists; experts get only critical alerts. This tailored approach respects experience while ensuring consistency.
Modernizing Legacy Systems, Not Replacing Them
Many manufacturers operate equipment older than the concept of AI. Retrofitting these machines with IoT sensors and edge computing modules could help bridge the gap in the future. Vibration sensors on a 20-year-old lathe, for example, feed data to AI models that predict bearing wear. Edge devices process this data locally, sending only actionable insights to central systems.
Data security remains paramount. On-premise edge processing ensures proprietary parameters—like alloy formulas or machining tolerances—never leave the facility. This balance of innovation and caution makes AI adoption feasible for regulated industries like aerospace or pharmaceuticals.
Sustainability Through Precision
AI’s environmental impact is often an afterthought, but it’s profound. Optimizing furnace temperatures in real time could help reduce fuel use without compromising output as AI adoption progresses. Analyzing material waste patterns reveals design tweaks that maintain strength while using fewer resources.
These gains aren’t incidental. They stem from AI’s ability to model thousands of variables—energy costs, carbon footprints, scrap rates—and find efficiencies humans might overlook.
Building Trust Through Transparency
AI’s success hinges on workforce buy-in. Workers need clarity on how tools augment—not audit—their roles. Pilot projects should start with narrow, high-impact use cases:
- Reducing unplanned downtime on a critical machine.
- Cutting material waste in a high-defect process.
- Streamlining changeovers on a bottleneck line.
INDUSTRIOS is exploring modular AI tools that could integrate with existing MES or SCADA systems. Our solutions prioritize interoperability, ensuring upgrades enhance rather than upend current workflows.
Final Thoughts on Future of AI on the Shop Floor
Future AI applications on the shop floor may break down silos between departments. For example, a quality defect detected in final assembly might one day trigger automatic adjustments in upstream machining parameters using AI on the shop floor. Predictive maintenance alerts powered by AI on the shop floor could reschedule non-urgent repairs to align with planned downtime, minimizing disruption.
For manufacturers, the future is about harnessing AI on the shop floor to build smarter facilities where wisdom scales, waste shrinks, and expertise outlasts retirements. This includes bridging legacy systems and modern safety demands through ongoing research and development, ensuring AI on the shop floor drives efficiency and innovation.